Introdução
Vivemos num mundo onde tudo gera dados. Cada clique, compra, conversa ou cadastro se transforma em fragmentos digitais que crescem em velocidade exponencial.
Segundo uma pesquisa da Exploding Topics, mais de 328 milhões de terabytes de dados são criados todos os dias. Isso equivale a cerca de 328 milhões de HDs de 1 terabyte sendo preenchidos diariamente — um volume tão gigantesco que escapa à nossa capacidade humana de interpretação.
Mas o volume, por si só, não significa vantagem competitiva. Dados brutos são como petróleo recém-extraído: ainda não têm forma, nem valor direto. Só viram combustível real quando passam por um processo de transformação. E é aí que muitas empresas travam, sem saber por onde começar ou o que exatamente extrair desse “oceano bruto”.
Neste artigo, vamos dar o primeiro passo: entender, de forma simples e prática, como transformar dados em inteligência real. Você vai descobrir o que isso significa na prática, por que isso importa para o futuro do seu negócio e como essa transformação viabiliza o uso da inteligência artificial (IA) com mais segurança, velocidade e clareza.
Seja qual for o tamanho da sua empresa, essa jornada começa com uma pergunta: “o que seus dados estão tentando dizer?”.
Vamos descobrir!
Transformação de dados: do petróleo digital ao combustível da IA
Se dados brutos são o “novo petróleo”, a transformação de dados é o processo que os torna utilizáveis — algo comparável a transformar o óleo bruto em combustível de qualidade, pronto para movimentar sistemas inteligentes com eficiência e segurança.
No contexto da inteligência artificial, essa transformação é o que separa iniciativas que apenas reagem, daquelas que antecipam, aprendem e evoluem. Porque não basta coletar dados: é preciso tratá-los, organizar e dar sentido a eles. Só assim é possível gerar inteligência de verdade.
Transformar dados, na prática, significa reunir informações espalhadas em diferentes sistemas (como planilhas, CRMs, ERPs, e-commerces, bases públicas, etc.), e trabalhar essas informações para que façam sentido quando colocadas lado a lado. Isso envolve padronizar, limpar, conectar e estruturar os dados de modo que possam ser usados com confiabilidade, inclusive em aplicações de IA.
Esse é um passo decisivo para qualquer empresa que busca agilidade nas decisões e previsibilidade nas ações. E o mais importante: esse processo não precisa ser complexo ou inacessível. Com as tecnologias certas, a transformação de dados pode ser automatizada e contínua, deixando de ser um gargalo e se tornando uma vantagem competitiva real.
Mas afinal, por que isso se tornou tão urgente agora? O que mudou no cenário atual que fez da transformação de dados uma prioridade estratégica para empresas de todos os portes? É o que veremos a seguir.
A importância da transformação de dados na era da IA
A inteligência artificial não funciona com qualquer dado: ela depende de uma base sólida, confiável e bem estruturada. Se os dados chegam incompletos, desconectados ou duplicados, a IA perde eficiência e, pior ainda, pode gerar respostas distorcidas. É como tentar construir um raciocínio lógico com informações desencontradas; o resultado dificilmente será coerente.
É por isso que a transformação de dados deixou de ser um diferencial técnico e passou a ser uma condição básica para quem deseja usar IA de forma estratégica. Mais do que uma questão de tecnologia, é uma escolha sobre como as decisões serão tomadas a partir de agora: com base em dados claros ou em suposições vagas?
Assim, empresas que dominam seus dados conseguem prever tendências, automatizar rotinas, reduzir riscos e responder com agilidade às mudanças do mercado. E, ao contrário do que se imagina, essa capacidade não está limitada às grandes corporações. O que faz a diferença é o processo — e é justamente ele que vamos detalhar a seguir.
O processo de refinamento: da coleta à análise
Transformar dados não é uma etapa única, mas uma jornada contínua que passa por cinco fases principais:
- Coleta: identificar e reunir dados de diferentes fontes, como planilhas, sistemas, bancos, APIs, CRMs, ERPs, entre outros;
- Padronização: alinhar formatos, campos, nomenclaturas. É aqui que muitos problemas de duplicidade ou incompatibilidade são resolvidos;
- Qualidade e limpeza: eliminar inconsistências, dados duplicados, entradas incompletas ou obsoletas;
- Estruturação e integração: organizar os dados em modelos que permitem cruzamentos, relacionamentos e análises mais inteligentes;
- Análise e ativação: com a base preparada, os dados ganham vida, seja para alimentar sistemas de IA, dashboards ou para embasar decisões humanas com mais clareza.
Cada uma dessas etapas é essencial para garantir que o que entra no sistema seja, de fato, um ativo valioso, e não apenas “volume” sem contexto.
E agora que você entendeu o porquê e o como da transformação, a próxima pergunta é inevitável: o que sua empresa realmente ganha com isso? Vamos descobrir.
Benefícios da transformação eficiente de dados para empresas
Transformar dados não é só uma etapa técnica, e sim, uma virada estratégica. Quando feita com eficiência, essa transformação permite que os dados deixem de ser um repositório estático e passem a impulsionar decisões, automatizar processos e revelar oportunidades.
É como sair de um carro sem painel para um modelo de alta performance, com todos os dados em tempo real no display: velocidade, rota, combustível, temperatura. A diferença é que, nos negócios, esses indicadores apontam para desempenho financeiro, comportamento de clientes, gargalos operacionais e muito mais.
Empresas que dominam essa jornada conseguem:
- Agir com previsibilidade: usando dados históricos e contextuais para antecipar demandas e reduzir riscos;
- Tomar decisões com mais segurança: baseadas em evidências, não em achismos;
- Ganhar tempo e eficiência: eliminando tarefas repetitivas com automações inteligentes;
- Aumentar a competitividade: com uma leitura clara do mercado e da operação;
- Personalizar ofertas e experiências: cruzando dados de comportamento com histórico e preferências;
- Liberar o potencial da IA: alimentando algoritmos com dados confiáveis e estruturados.
Tudo isso gera uma cultura mais ágil, mais analítica e menos vulnerável à incerteza, exatamente o que diferencia empresas que apenas reagem daquelas que lideram.
E se os ganhos são claros, como colocar tudo isso em prática? No próximo tópico, mostramos o que sua empresa precisa para implementar esse processo com assertividade. continue acompanhando!
Implementando a transformação de dados na sua empresa
Como mencionamos, transformar dados com eficiência não é uma missão exclusiva de grandes corporações, e com equipes robustas de tecnologia. Cada vez mais, esse processo tem se tornado acessível, especialmente quando há clareza sobre os objetivos e as perguntas que precisam ser respondidas.
A primeira etapa não está nas ferramentas, mas no entendimento do seu próprio caminho. Assim como um piloto conhece cada curva do circuito antes da corrida, sua empresa precisa identificar quais são os dados mais relevantes, onde eles estão e o que precisa ser respondido com base neles. Com isso em mente, o próximo passo é estruturar um fluxo que permita:
- Coletar os dados certos, das fontes certas;
- Integrar essas informações sem criar ruído;
- Transformar e organizar os dados para uso contínuo;
- Distribuir esses dados para as pessoas e sistemas que vão utilizá-los.
Esse fluxo não precisa ser manual, lento ou complexo. É aqui que entram as ferramentas certas; confira.
Ferramentas e tecnologias essenciais para o processo
Uma transformação de dados eficiente se apoia em tecnologias que automatizam o caminho do dado, desde sua origem até a geração de valor. Entre os recursos mais importantes estão:
- Plataformas de integração (iPaaS): conectam dados de diferentes fontes (planilhas, CRMs, ERPs, bancos, APIs) com rapidez e flexibilidade;
- Ambientes de transformação (ETL/ELT): responsáveis por organizar, limpar, padronizar e preparar os dados para uso real;
- Estruturas de armazenamento modernas (data lake ou lake house): armazenam os dados em diferentes níveis de preparo, viabilizando análises mais rápidas e confiáveis;
- Linguagens flexíveis de manipulação: como SQL e JavaScript, que permitem ajustes finos no caminho dos dados, com agilidade e controle;
- Painéis e visualizadores: ferramentas como Power BI, Metabase e dashboards personalizados transformam dados em leitura visual clara e acessível.
Essas tecnologias permitem que a transformação de dados aconteça de forma integrada, segura e escalável, sem exigir do negócio uma frota inteira de especialistas para pilotar o processo.
Mas, como toda jornada de inovação, implementar transformação de dados também traz desafios. No próximo tópico, abordaremos os principais pontos de atenção e como superá-los de forma estratégica.
Desafios e considerações ao trabalhar com transformação de dados
Transformar dados em ativos estratégicos não acontece de forma automática. Como qualquer sistema complexo, é preciso afinar engrenagens, testar limites e estar atento a pontos críticos que podem comprometer todo o percurso.
A seguir, mostramos os desafios mais comuns nessa jornada e o que considerar desde o início para garantir tração e consistência nos resultados!
Privacidade e segurança dos dados
Em um cenário onde as empresas lidam com volumes crescentes de informações sensíveis, a segurança é o primeiro componente que precisa estar sob controle. Não basta acelerar: é preciso garantir que o freio funcione, que os dados estejam protegidos por camadas de segurança e conformidade legal, como exige a LGPD (Lei Geral de Proteção de Dados).
Isso inclui práticas como criptografia, controle de acesso, anonimização e armazenamento seguro. Ou seja, a IA só pode operar com confiança quando os dados estão protegidos por um ambiente robusto e blindado.
Como gerenciar grandes volumes de informação
Dados vêm de todos os lados: ERPs, CRMs, planilhas, APIs, bancos públicos e mais. Gerenciar esse volume exige uma estrutura pensada para alta velocidade e estabilidade. É aqui que entram soluções como os data lakes e lake houses, que atuam como centros de abastecimento bem organizados — separando o dado cru do dado pronto para uso, sem travar o sistema.
Com isso, é possível manter a operação fluida, sem gargalos ou sobrecarga de processamento, mesmo quando o volume de dados aumenta.
A importância de profissionais qualificados na análise de dados
Por mais que a tecnologia evolua, nenhum sistema roda sozinho sem um bom piloto. Profissionais qualificados fazem a diferença ao interpretar contextos, validar a qualidade da informação e direcionar os dados para decisões mais inteligentes.
Eles são os responsáveis por transformar números em narrativas estratégicas, e garantir que os dados, uma vez refinados, realmente gerem impacto no negócio.
Mudança de mentalidade e cultura organizacional
Adotar uma cultura orientada por dados é como mudar o estilo de pilotagem: exige treino, consistência e clareza de propósito. Não se trata apenas de ferramentas, mas de pessoas que confiam nos dados para decidir, aprender e ajustar o rumo com base em evidências, não em suposições.
Quando essa cultura se consolida, o dado deixa de ser apenas um relatório no fim do mês, e passa a ser um ativo que guia o dia a dia da empresa.
Superar esses desafios é o que garante estabilidade e escala. E, com a estrutura certa no lugar, agora, é hora de olhar para frente: o que vem a seguir no papel dos dados dentro da inteligência artificial? Confira.
O futuro da transformação de dados e seu impacto na IA
Nos próximos anos, o avanço da inteligência artificial não será mais medido apenas pela capacidade de responder rápido, mas pela qualidade do aprendizado que ela é capaz de absorver em tempo real — e isso está diretamente ligado à forma como os dados são transformados no dia a dia da operação.
Hoje, empresas mais maduras já começam a incorporar camadas de IA dentro do próprio pipeline de dados. Isso significa que processos como padronização, correção, enriquecimento e categorização passam a ser feitos automaticamente, sem depender de codificação ou ajustes manuais. A IA atua antes mesmo da análise: ela organiza, alerta, antecipa.
Segundo a McKinsey, 72% das empresas já utilizam algum nível de IA, o que mostra que a adoção cresceu, mas ainda falta preparo na base. Esse cenário abre espaço para um movimento decisivo: a adoção de modelos generativos privados, treinados com dados internos e protegidos por ambientes controlados.
Em vez de usar uma IA genérica treinada com conteúdo externo, essas empresas desenvolvem agentes inteligentes capazes de responder com base em contratos, manuais técnicos, históricos de atendimento ou qualquer outra fonte estratégica do negócio.
Não se trata apenas de eficiência, mas de construir uma inteligência que respeita o contexto e a confidencialidade da operação. O resultado? Menos dependência de dados públicos, mais precisão nas respostas e maior controle sobre os modelos que realmente geram valor.
Esse futuro já está em construção. E quem começa agora a estruturar os dados com visão estratégica, coloca sua empresa à frente no jogo da inteligência.
No próximo bloco, mostramos como a Skyone já entrega esse cenário na prática!
Como a Skyone potencializa a transformação de dados na sua empresa
Na Skyone, não acreditamos em soluções genéricas. Sabemos que cada empresa tem um ponto de partida diferente, e é exatamente por isso que nossa plataforma foi pensada para se adaptar aos cenários mais diversos, sem travar, sem complicar, sem exigir uma revolução interna.
Ao longo dos anos, percebemos que o verdadeiro desafio não está apenas em integrar sistemas, mas em dar fluidez à jornada dos dados, da origem até a aplicação prática. Por isso, criamos uma estrutura que elimina ruídos, automatiza etapas e entrega visibilidade em tempo real sobre tudo o que está sendo transformado.
Na prática, isso significa que conseguimos:
- Ler dados diretamente de planilhas, sistemas legados, bancos SQL e fontes externas, sem depender de codificações manuais a cada nova entrada;
- Aplicar lógicas de transformação em JavaScript e JSONata com flexibilidade, como se cada dado passasse por um ajuste fino sob medida antes de chegar à IA;
- Operar tanto em ambientes de nuvem quanto locais, respeitando o que cada cliente precisa em termos de compliance, privacidade e performance;
- E manter tudo isso conectado em um único lugar, com governança, rastreabilidade e controle total.
Nosso papel é garantir que os dados da sua empresa circulem como devem: sem fricção, com clareza, e prontos para gerar inteligência real. Nossa plataforma faz o trabalho pesado nos bastidores, enquanto você e sua equipe focam em usar os dados como ativo estratégico.
Quer tirar isso do papel e ver como se aplica à sua operação? Fale com um especialista da Skyone. Estamos prontos para te ajudar a transformar dados em decisões com muito mais autonomia, velocidade e escala!
Conclusão
Transformar dados não é apenas um movimento técnico: é um amadurecimento estratégico. Ao longo deste artigo, vimos que dados brutos não têm valor por si só. Eles precisam ser extraídos, organizados, refinados e ativados para que possam gerar decisões mais ágeis, respostas mais precisas e inteligência real em aplicações de IA.
Ficou claro que o desafio não está apenas na quantidade de informação disponível, mas na capacidade de estruturar essa informação com consistência, segurança e contexto. E que esse processo não depende de projetos gigantes ou estruturas complexas: depende de visão, intenção clara e ferramentas que tornem essa transformação fluida.
À medida que a inteligência artificial avança, a forma como tratamos os dados se torna ainda mais determinante. Quem quiser acelerar, com estabilidade e controle, precisa garantir que o “motor” dos dados esteja limpo, bem calibrado e pronto para responder com eficiência. Foi com essa visão que organizamos este conteúdo: para ajudar você a enxergar os dados como um ativo vivo, não como um arquivo estático.
Quer continuar explorando como dados e IA podem se traduzir em vantagem real nos negócios? Leia também nosso artigo “IA para negócios: como a inteligência artificial pode transformar sua empresa”!
FAQ: perguntas frequentes sobre como transformar dados brutos para IA
Seja para quem está começando a explorar o universo da inteligência artificial (IA), ou para quem já entende a importância dos dados, surgem sempre as mesmas dúvidas iniciais: “minha empresa está pronta?”, “Preciso de uma estrutura robusta?”, “Isso serve para negócios menores?”.
Aqui, reunimos as perguntas mais comuns, e as respondemos com objetividade, clareza e aplicabilidade real.
1) Empresas de pequeno porte podem se beneficiar da transformação de dados?
Sim. Negócios de todos os tamanhos podem se beneficiar da transformação de dados, especialmente os menores, que ganham agilidade e inteligência sem precisar de estruturas pesadas. Com ferramentas acessíveis e automações simples, é possível integrar informações de planilhas, CRMs ou ERPs e começar a tomar decisões mais assertivas, com base em evidências. O segredo está em começar com clareza sobre quais dados são mais relevantes e qual o objetivo de transformá-los.
2) Como saber se minha empresa está pronta para adotar a transformação de dados?
Você não precisa ter tudo organizado para começar, mas precisa saber o que quer descobrir com os dados. Se sua empresa já tem processos digitalizados (em CRMs, planilhas, plataformas de vendas, etc.), e enfrenta dúvidas como: “por que os resultados variam?”, “onde estamos perdendo oportunidades?”, “o que podemos prever melhor?”, então já existe um ponto de partida. A transformação de dados serve justamente para dar clareza ao que hoje está disperso. O mais importante é ter um problema ou objetivo claro. O resto pode (e deve) ser construído ao longo do caminho.
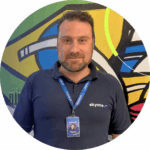
Theron Morato
Especialista em dados e chef de cozinha nas horas vagas, Theron Morato traz um olhar único sobre o universo dos dados, combinando tecnologia e gastronomia em metáforas irresistíveis. Autor da coluna “Data Bites” no LinkedIn da Skyone, ele transforma conceitos complexos em insights saborosos, ajudando empresas a extraírem o melhor de seus dados.
Autor
-
Especialista em dados e chef de cozinha nas horas vagas, Theron Morato traz um olhar único sobre o universo dos dados, combinando tecnologia e gastronomia em metáforas irresistíveis. Autor da coluna "Data Bites" no LinkedIn da Skyone, ele transforma conceitos complexos em insights saborosos, ajudando empresas a extraírem o melhor de seus dados.